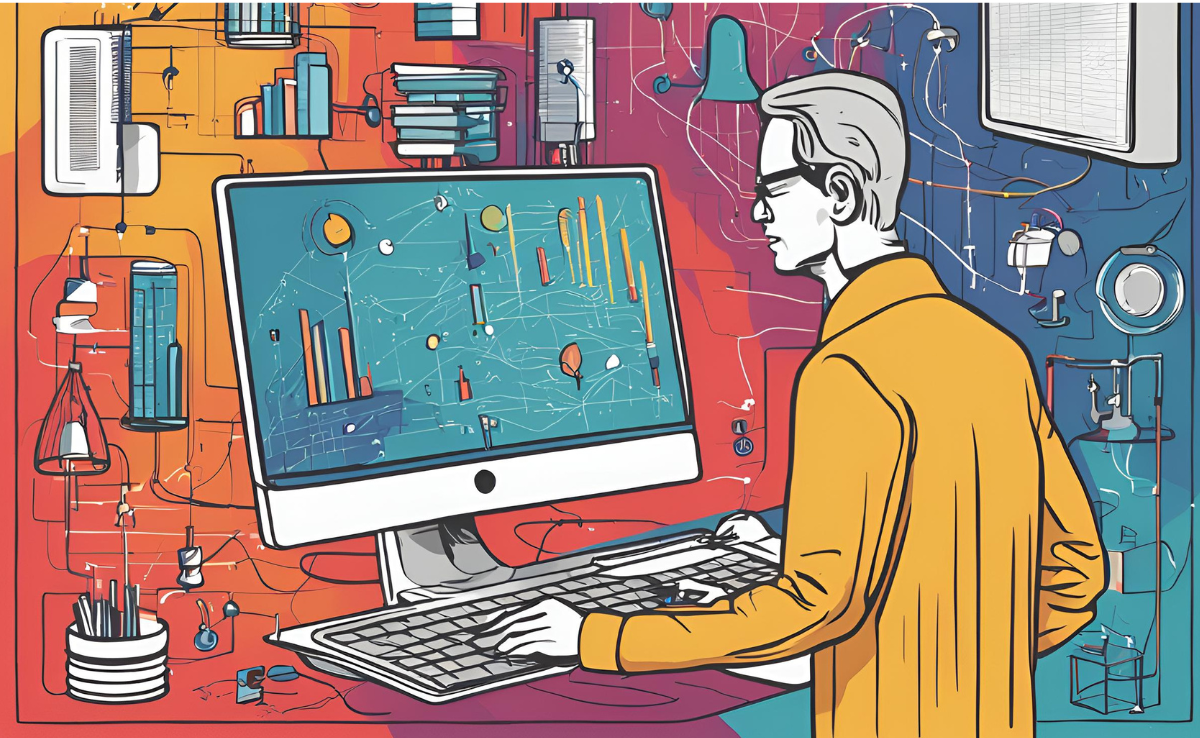
For the past five years, NGP Capital, the independent investment fund of Finnish telecommunications and electronics maker Nokia, has quietly developed its own artificial intelligence-driven platform for scouting potential investments.
In 2019, a couple of years before the hype around large language models emerged with the release of OpenAI’s ChatGPT in November 2022, NGP Capital hired a data scientist, Atte Honkasalo, to develop an in-house proprietary analytics system to scout for investments.
An AI engine was added to the model in 2020, which distils data pulled from the web, matching it to the fund’s investment thesis and growth criteria. Through the platform, the team now monitors two million private technology companies with a focus on North America and Europe.
“We wouldn’t have known about these companies had we not had them in our system.”
Paul Asel, founding partner, NGP Capital
The model has been “extremely useful” in the investment team’s evaluation of deals, says Paul Asel, founding partner at NGP Capital. “We have sourced many deals from that. We wouldn’t have known about these companies had we not had them in our system.”
Supporters of AI say these kinds of models that NGP Capital has developed could signal a new dawn for VC investing. Artificial intelligence could bring a more analytical layer to venture capital investments, which have so far been based more on gut instinct and high-risk decision-making.
“We are going to experience a tremendous shift in the investment industry,” says Matias Collan, CEO of ACE Alternatives, a fund administration services company. “You have investment analysis tools, portfolio modelling tools, valuation tools, risk analysis tools. And AI tools that enable you to negotiate your investment transaction-related documentation.
“It is mind boggling that 10 years ago that would have been a dream but now it is a reality,” says Collan.
While financial VC firms are using AI across a variety of functions, from automating responses to founders to deal sourcing, corporate venture capital units, aside from firms like NGP Capital, appear to be way behind the curve.
CVCs have to play catch-up
“From the couple of dozen CVCs that we work with, there hasn’t even really been a discussion about AI, period,” says Kevin Ye, managing partner at Mach49, an advisory firm that helps companies build and invest in ventures.
One reason for this is the lack of maturity of CVCs. Many don’t have the internal processes or their own data for AI-based investing to work.
“If you want to use AI for something like deal sourcing, you need to have a good sense of your criteria and have your own data and historical kind of trends that you have done yourself. That can be tough for a lot of CVCs,” says Ye.
Another hindrance is CVCs’ reliance on their parent companies’ IT systems. Most CVCs still invest off the parent’s balance sheet and have neither the permission nor the resources to start using another purpose-built IT platform.
“I would not want to do this within a corporate environment.”
Paul Asel, NGP Capital
Asel, of NGP Capital, says he would not have even tried to build an AI-driven software platform if his investment firm was tied to the parent company’s own systems. “I would not want to do this within a corporate environment. It doesn’t mean a CVC shouldn’t do it. But I would not want this to be part of a corporate IT system,” says Asel.
Developing an AI-driven platform also requires a lot of money. While Asel did not go into exactly how much the firm has spent on its system, he says only large investment units should consider this. NGP Capital is one of the larger CVC funds with $1.7bn in assets under management. “Only a firm that has sufficient scale could contemplate something of this magnitude,” says Asel.
As CVCs become more professionalised and independent from their parent corporations, it is possible corporate investors will use AI more for deal scouting. But proponents of the technology stress that investors need to be aware of shortcomings in the output of the models.
Tackling the problem of bias
Models pulling on inaccurate data is an obvious flaw. NGP Capital’s AI platform draws on data from industry vendors as well as publicly accessible data such as company websites. Honkasalo, vice president of data and analytics at NGP Capital, says the team does a lot of data quality monitoring to check for errors.
The model also does not pull personal data on startup founders such as gender and age to avoid bringing in explicit bias. But even this is not failsafe. Honkasalo says AI models can still learn bias without personal data inputs. “They are able to learn [bias] somehow from other variables that seem harmless,” he says. “At the end of the day, the most important thing is to be aware of that risk.”
Despite this, Asel says the model mitigates bias by serving as first approval of companies that the team should consider. He is more concerned about bias coming in during the investment process. “There’s all kind of biases that introduce themselves when we meet the team. This actually reduces bias for us because it serves as a first pass, and then as we go through the process it also gives us a more distance perspective,” says Asel.
Others are more wary about the risks of AI in reinforcing bias in investment decisions. Clint Korver, managing director at US VC firm Ulu Ventures, is worried that AI will reinforce bias in the data rather than highlight it. “The AI is basically reinforcing the existing perspective of the data that it has been trained on,” he says.
Venture capital is one of the most biased asset classes in existence, with only 1% of capital going to black and Latinx founders.
“Venture capital is literally decades behind almost every other asset class in terms of sophistication in collecting data, evaluating risks and building portfolios.”
Clint Korver, Ulu Ventures
Korver set up his investment firm with the intention of trying to limit bias in decision-making by having a diverse team that includes women and people of colour. He adds that venture capital “is literally decades behind almost every other asset class in terms of sophistication in collecting data, evaluating risks and building portfolios.”
Korver does see some potential in using AI to source deals if it can identify outliers, for example, although he questions whether models trained on this can be called AI. “If a company’s Twitter mentions go up by 100% in a month, I want to know about that. That is a stretch to call that AI because somebody just sends a rule, and they are just looking for it.
“There is a lot you can do with averages and outliers. It is just kind of being smart about the data that doesn’t require neural nets and all that fancy stuff,” says Korver.
For now, AI appears to be more of a game changer for saving time summarising documents or automating certain repetitive tasks. But more sophisticated models for deal sourcing that inform investment decisions look to be on the horizon for CVCs with plenty of money to spend.