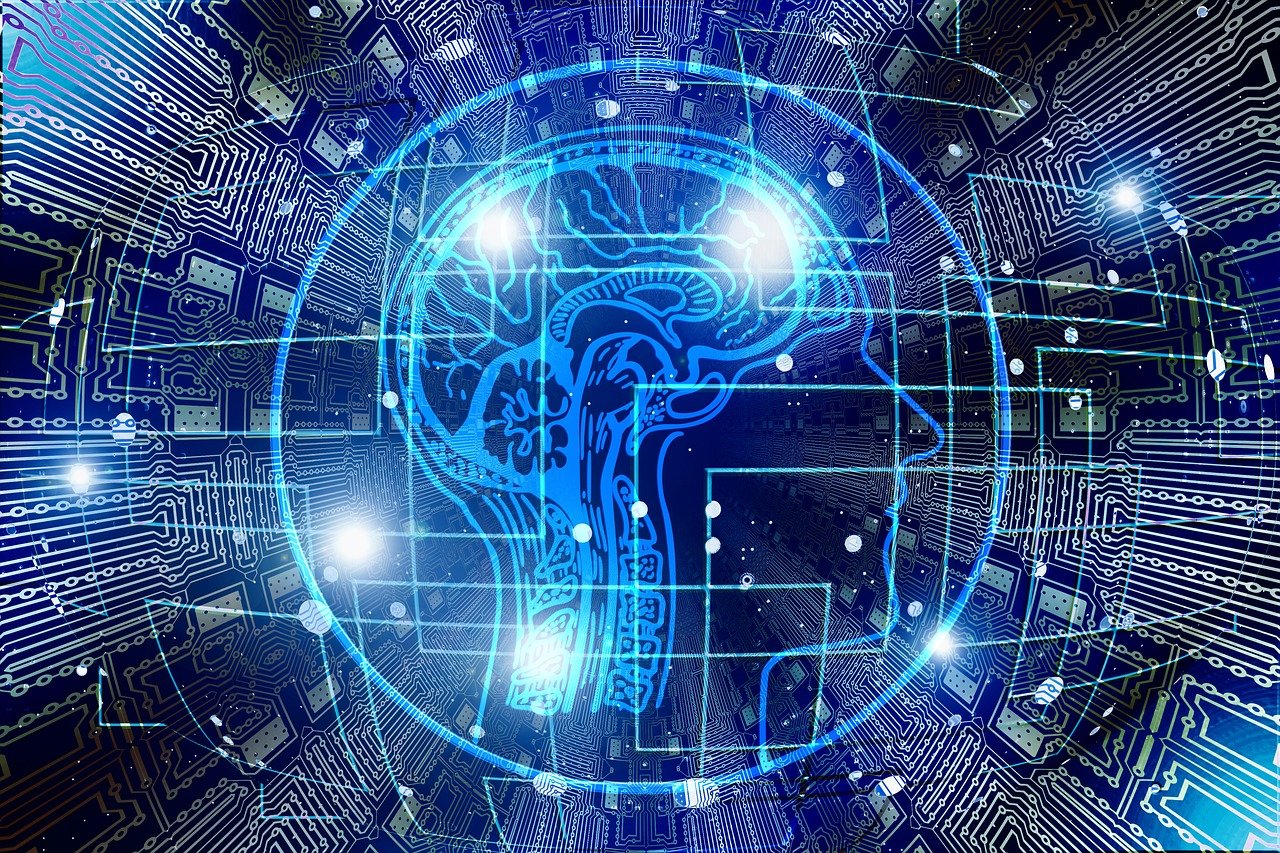
ChatGPT, the large language model chatbot developed by OpenAI, started a frenzy of investment when it was released in late 2022, with $7.6bn spent since the start of this year on funding startups developing generative AI-related technologies, according to Pitchbook. Several large funds have launched this year that focus specifically on generative AI technology.
But many corporate investors are bemused about how best to invest in the technology, which still has one fundamental problem for them: a lack of good quality data.
“The problem is data is mostly not available if you want to build your own model,” says Ilja Aizenberg, principal at M Ventures. “Those general-purpose models are not suitable for many industry-specific use cases. For this, you need to make specific models and specific data to train those models.”
Corporate investors have little use for general-purpose large language models like ChatGPT, which have been trained on web-crawler accessible data that hasn’t been validated or checked for accuracy. But the data to build more industry-specific models is not readily available. Corporate investors are also concerned that they need to ensure models are built on data that maintain users’ privacy, which is especially critical in industries such as healthcare and financial services.
“The problem is data is mostly not available if you want to build your own model.”
Ilja Aizenberg, principal at M Ventures
M Ventures isn’t a newcomer to AI. Like many corporate investors the investment arm of German science and technology company Merck had invested in artificial intelligence startups long before this latest wave of hype around AI began. Its startup portfolio includes Celestial.AI and MemryX, which aim to boost computing power necessary for AI and big data processing. The team is now taking a hard look at how generative AI could be a strategic fit — but so far it is a struggle.
Aizenberg points to the lack of good data in the chemical industry as an example. This sector’s data is biased because it is based on research that has mostly positive outcomes. It is the same for many research-based sectors.
Other corporate investors say they have yet to see a good business case for investing in generative AI. In the energy sector, for example, reliable data is so sparse that it is difficult to build large language model that could come up with reliable solutions to the industry’s problems. “Everyone thinks we have enough data. But we don’t have the data organised in any way that can be efficiently used,” says Patrick Walsh, director of National Grid Partners, the corporate VC arm of UK-based utility National Grid.
One solution is to create synthetic data. This is data that is processed and cleaned so it can be used for industry-specific solutions. “We will see companies addressing this issue through synthetic data but also through automation of generating new data from R&D,” says Aizenberg.
In the meantime, corporate investors are often sticking to simple uses for generative AI. For example, Vision Ventures, the corporate venture arm of Mexican ophthalmology-based pharmaceutical company Laboratorios Sophia, is considering investing in a startup developing technology that generates answers to patient questions about their eye health.
Addy Liñán Segura, principal of Vision Ventures, says the unit has also identified a strategic need of its parent company to access patients at earlier stages of their eye diseases. “How we extract strategic value is part of the discussion,” she says of the unit’s investing in AI. “There are a lot of AI options. But there should be something else other than just the technology.”
Valuations are too high
The large interest in generative AI has led to startups becoming overvalued. Financial VCs have jumped into investing in generative AI startups to ensure access to deal flow rather than relying on the soundness of startups’ business models, say investors.
“From an investment perspective, there are so many questions when investing in such companies around foundational models, where there is no clear business model. Most of these funds have gone into investing in the team and pre-seed rather than business model and logic. This has worked well in the past for VC,” says Aizenberg.
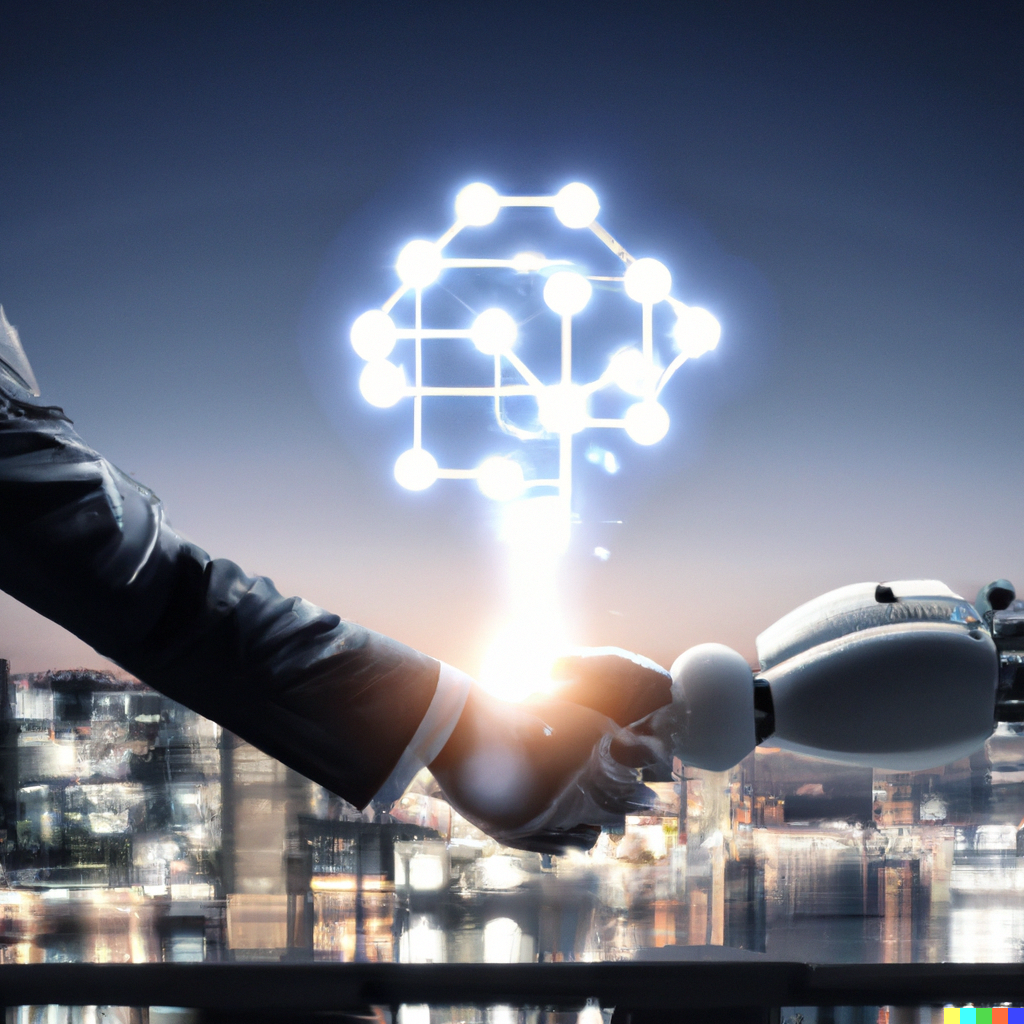
“I think there are currently too many startups chasing some of these early and obvious generative AI deployment areas,” says Walsh. “Similarly, the deployment of large-scale search-enhancement tools seems limited to the current largest cloud service providers. Deployment of generic office suite enhancements likely will see Microsoft and Google take most of the white space in those areas,” he says.
National Grid Partners has invested in several companies that collect data through sensors and use advanced data analytics and machine learning rather than generative AI. Examples include Exodigo, which uses sensors for underground mapping to support utility construction and underground repairs; Tomorrow.io, which deploys sensors and satellites with proprietary models to predict weather; and Line Vision, which uses imaging and vision software to monitor transmission lines.
“There are too many startups chasing some of these early and obvious generative AI deployment areas.”
Patrick Walsh, director of National Grid Partners
The biggest value Walsh sees in generative AI specific to the energy sector is in mission critical immersive augmented reality, where utilities generate virtual scenarios for disaster or recovery planning. In this so-called digital twin space, Walsh sees generative AI playing a role in providing advice and instructions in virtual worlds.
This becomes important to utilities such as National Grid as they move into virtual modelling of the grid to manage operations as well as plan for new infrastructure such as transmission lines. “The incremental improvement of smarter AI assistants in these environments will provide a noticeable jump in usability and functionality for expert users,” he says.
But, despite claims that generative AI could be bigger than the internet, the technology isn’t going to solve the biggest issue facing the energy sector: the transition to net zero that will require massive investment in infrastructure and new generation.
“Those kinds of mega tasks are where we are focusing our efforts. Do I see generative AI solving those? It is a good research assistant, but I don’t see it as solving those problems,” says Walsh.
Others caution that strategic investors should not overestimate the impact of generative AI in the short term. “Don’t get overexcited,” says Owen Lozman, managing director of M Ventures. “We are still early in this space. Focus on where you can really drive strategic value in your businesses. Leverage what is out there.”