Everyday media is full of news of privacy breaches, algorithmic biases and AI oversights. In the past decade, public perceptions have shifted from a state of general obliviousness, to a growing recognition that AI technologies, and the massive amounts of data that power them, pose very real threats to privacy, accountability, and transparency, and to an equitable society. The Ethical AI Database project (EAIDB) seeks to generate another fundamental shift – from awareness of the challenges, to education of potential solutions – by spotlighting a nascent and otherwise opaque ecosystem of startups that are geared towards shifting the arc of AI innovation towards ethical best practices, transparency and accountability.
EAIDB, developed in partnership with the Ethical AI Governance Group, presents an in-depth market study of a burgeoning ethical AI startup landscape geared towards the adoption of responsible AI development, deployment and governance.
We identify five key subcategories, then discuss key trends and dynamics.
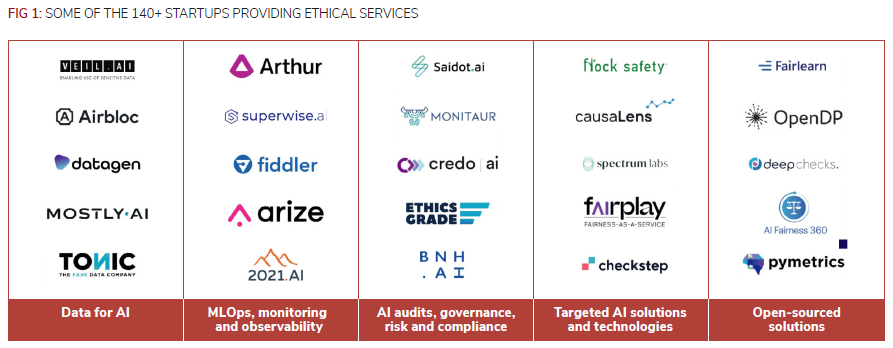
Motivation
The concept of ethical artificial intelligence (AI) is quickly building momentum, with startup executives developing AI-first solutions, enterprise customers deploying them and VC and CVC investors that are financing them, as well as for end users consuming them, academics researching them and policymakers seeking to regulate them. The sheer volume of companies identified as “ethical AI companies” in this study pays testament to this emerging reality.
While ESG has traditionally focused on “do no harm” versus “do good,” ethical AI businesses, for the purposes of this research, include solutions that either remediate the pitfalls of existing AI systems (“do no harm”) or that leverage AI to address a broader societal good (“do good”). The space has seen very significant growth in the past five years.
The motivation behind this market research is multidimensional:
n Investors are increasingly seeking to assess AI risk as part of their comprehensive profiling of AI companies. EAIDB provides transparency on the players working to make AI safer and more responsible.
n Internal risk and compliance teams need to operationalise, quantify and manage AI risk. Identifying a toolset to do so is critical. There is also an increasing demand for ethical AI practices, as identified in IBM Institute for Business Value’s report.
n As regulators cement policy around ethical AI practices, the companies on this list will only grow in salience. They provide real solutions to the problems AI has created.
n AI should work for everyone, not just one portion of the population. Enforcing fairness and transparency into black box algorithms and opaque AI systems is of the utmost importance.
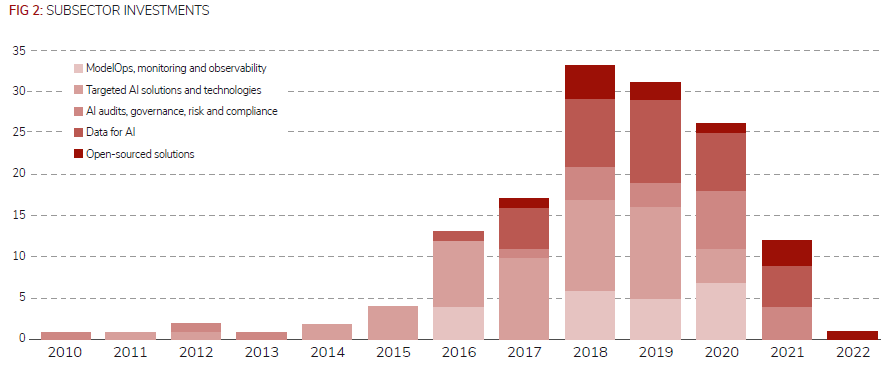
Category 1: Data for AI
Description
Companies in this category provide specific services to maintain data privacy, detect data bias early, or provide alternative methods for data collection/generation to avoid bias amplification later in the machine learning lifecycle. A large proportion of companies in this category specialise in synthetic data: essentially, generating a new, artificial dataset that is statistically guaranteed to behave similarly to the old one. However, because this data no longer refers to real people or true information, there are no privacy concerns. These companies compete on how similar their synthetic sets are relative to the original datasets and how flexible their solutions are (for example, can the product handle both unlabelled and labelled data?). Other subcategories include data sharing (data permissions, safe transfer, etc.), data privacy (anonymisation, differential privacy, etc.) and data sourcing (representative samples, minority amplification, etc.). Companies generally deliver their services through APIs or CLIs.
Trends and dynamics
Some players in this space are experts in one area of synthetic data. Datagen, for example, focuses on facial data by allowing datasets to contain diverse skin colours, hairstyles, genders and angles to minimise the risk of biased facial recognition technologies. “Sourcers”, such as co:census or Snorkel AI, base their products on ethical sourcing – the former amplifying voices and ensuring representative sampling via SMS and the latter performing fair, automatic labelling with data versioning and auditing services. Versioning and auditing in the context of bias will continue to play a large role in basic offerings provided by ethical AI companies. It might be that synthetic data companies will crowd each other out, since barriers to entry are low and only a few have superior products or have adopted niche data areas. Data sharing and privacy companies overlap with cybersecurity and secure computing, which are their main competition. What lacks in this space is “context-conscious data mining,” or a mining/sourcing platform that understands the context of the dataset and then assess potential bias concerns.
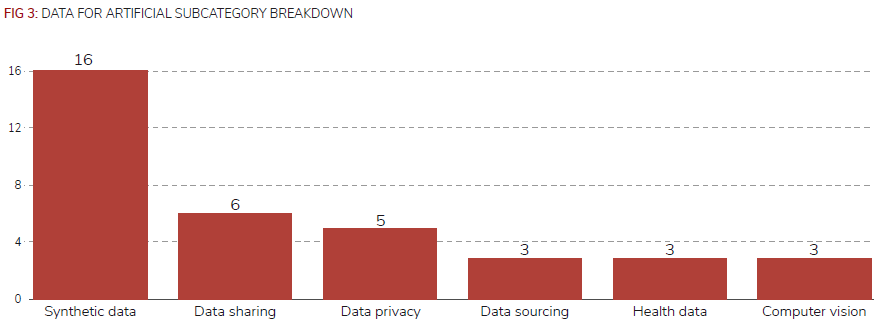
Category 2: ModelOps, monitoring and explainability
Description
Members of this category provide specific tooling to monitor and detect prediction bias (however, this may be defined in context). Usually self-defined as “quality assurance for ML”, these companies specialise in black box explainability, continuous distribution monitoring, and multi-metric bias detection. For the purposes of this study, MLOps companies that provide generic monitoring services fall outside of scope. Companies in this space are somewhat uniform in their offerings. MLOps platforms such as Fiddler or Arthur have features such as drift detection and bias detection/monitoring, but also touch on explainability (which is relatively low-effort to add in). Others in this space focus specifically on deep learning visibility. Many companies package themselves as traditional MLOps companies with the added upside of bias-related software. One increasingly interesting subtopic in the MLOps world is model versioning – which is very closely related to data versioning and overlaps with the next category, governance.
Trends and dynamics
The product differentiation between the top players in this category is relatively small. The incumbents in the MLOps space (Amazon, Datarobot, etc.) have quickly adopted bias-related technologies that present some form of competition, but specialised firms such as those in this category offer better integration and better monitoring products because these are their primary mode of business. Many constituents in this space have their own internal labs in which theory is applied to practice. This is rare in the corporate world, but the companies that constantly pay attention to the latest methods of bias detection and resolution and put them into use will surely cement themselves at the top. Bringing explainability to deep learning is a debatable proposition in its own right – Shapley values or LIME are disputed as proper explanatory methods. In regards to data involving humans, the use of deep learning will surely decline because these models present low interpretability. Regulatory compliance and risk analysis is, therefore, significantly more difficult. In this case, deep learning models are less desirable and platforms that focus on deep learning explainability, such as XaiPient are, by extension, less desirable. The theory around visibility in deep learning models will inform whether this subspace will wax or wane in demand.
Category 3: AI Audits and GRC
Description
Members of this group are usually specialist consulting firms or platforms that establish accountability/governance, quantify model and/or business risk, or simplify compliance for internal teams within AI systems. Consulting firms differentiate themselves according to experience and specialisation (for example, NLP, deep learning, etc.). Some, such as BNH.ai, are law firms that specialise in bias consulting. Top consulting firms (BCG, McKinsey, etc.) are main competitors and generally attract the most diverse clientele, though as larger and older companies they have different methodologies and expertise than smaller, more recent players. Software platforms tend to focus, instead, on increasing accountability and transparency by making AI models shareable with all stakeholders. Some allow for the “automation” of governance, such that risk is visible in the same way throughout the ML lifecycle. Documentation, reporting and other features are usually included alongside the main product. Certain niche companies, including EthicsGrade, apply objective frameworks to companies and assign “grades” to boost transparency among consumers, investors and regulators alike. Automated compliance is also a subcategory, in which companies such as Relyance AI enforce contractual compliance at the code level.
Trends and dynamics
The growth in AI audits and GRC companies has been constant over the past five years, thriving in part due to weaker policy and indecision among international governments regarding what constitutes best practices in AI. These firms are naturally the most flexible companies in EAIDB and are able to assist with various parts of the AI/ML lifecycle in a context-conscious way – an area where platforms will always struggle. However, as better metrics are created and the demand for automatic ML compliance increases, platforms such as Credo AI may begin eroding firms’ market shares. Automated GRC is still very general – there is still a lot of room for specialised, bias-conscious GRC solutions.
Category 4: Targeted AI solutions and technologies
Description
Targeted AI solutions encompasses AI companies that attempt to solve a particular ethical issue in a vertical. Sometimes describable as “a more ethical way to…”, these companies are usually contained within labels such as hiretech, insuretech, fintech, healthtech, etc. Targeted AI technologies refers more to general AI that is more horizontally integrated and vertically applicable. Some examples of common horizontals are toxic content detection, deepfake detection and ethical facial recognition. Due to a “hiring bias boom” in the mid-2010s, a majority of the companies in this category are hiretech companies. Pave is a benchmarking startup meant to provide tools to solve wage inequality, while Diversio can quantify and help improve DEI initiatives. Fair treatment in lending with companies such as FairPlay is also a hot topic (though fairness in finance has often been more regulated than in other fields). Other extremely niche companies, such as Flock Safety and Citibeats, touch on very interesting use-cases for responsible AI design and have relatively few competitors.
Trends and dynamics
As applications where ethical AI is necessary continue to increase, targeted AI solutions will become more popular. With the advent of deepfakes, for example, specialised companies involved with the ethical implications of the technology have been developed. The recent completion of the deciphering of the human genome might spur more AI companies related to biodata and genetic construction. A longer-term application space within healthtech might be related to ethical concerns regarding genetic data (not limited to privacy). There may be a new wave of insurance companies under insuretech that use alternative methods of calculating prices. Just Insure, for example, uses a customer’s driving habits to calculate what they should pay. This removes the need for background checks involving other types of data and therefore may reduce proxy bias. There is a lot of room for niche companies to grow in this category – there are verticals (such as ethical crime detection or ethical social media analytics) where there is almost no competition.
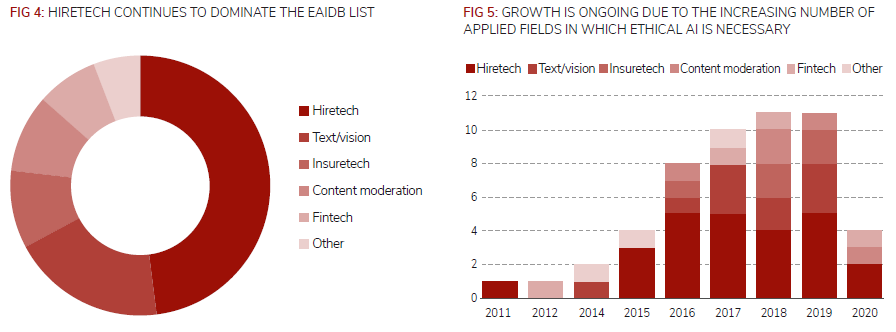
Category 5: Open-sourced technologies
Description
As the name suggests, this category contains fully open-sourced solutions, which are meant to provide easy access to ethical technologies and responsible AI. The companies behind these frameworks are usually not-for-profit (though some, such as Credo Lens, are), but their open-sourced technology is usually a good approximation of the cutting-edge in applied ethical AI research. Open-sourced tools play their own role within the startup ecosystem because they provide access to cheap tools that other, non-specialist firms can employ. Most open-source tools are concerned with privacy, bias detection and explainability. The shortcomings with this category are consistent with shortcomings of open-source in general: vulnerability to malicious users, lack of user-friendliness and lack of extensive support systems. However, they provide a baseline that companies need to constantly beat by providing more flexibility, better support and easier access to warrant the prices they charge.
Trends and dynamics
Companies usually cannot keep up with the rapid pace of theoretical development in a field as dynamic as algorithmic fairness. Open-sourced frameworks can, however, because there are few barriers to creating a GitHub repository and starting an open-source project. This category will always continue to grow because there are no competitors, as such. However, the open-source community is generally a good place to establish a baseline and identify aspects of ethical AI that are lacking from the for-profit world. Deepchecks, for example, is an open-sourced framework for ML model testing, with the ability to write tests for bias in vision and standard models. Its code repository boasts a high activity rate of three commits per day.
Ecosystem trends
As policy is created, refined and further defined, consulting firms may decline in popularity and MLOps/GRC platforms may rise due to the ability to programmatically enforce compliance given concrete metrics.
Incumbents in the space will start incorporating less effective versions of bias-related technology in an effort to keep their platforms viable in this new world of bias-conscious policy. They lack the specialty, expertise and first-mover advantage of these startups but have a well-established client base from which to draw.
The demand for ethical AI will perpetually increase because performing AI services correctly is domain-specific, context-specific and very unstable (i.e. always needs to be monitored and checked for quality). The “boom” for ethical AI is estimated to be somewhere from the mid-to late-2020s and will follow a curve similar to “ethics predecessors” such as cybersecurity in the late-2000s and privacy in the late-2010s. There will be a time in which policy, real case studies of AI gone wrong and new discovery of biased AI (and a genuine desire to fix it) nudges companies in the right direction – through fear or will – leading to large demand and more inclusions in EAIDB.
EAIDB is partnered with BGV and the Ethical AI Governance Group (EAIGG). Views expressed are the author’s only.de repository boasts a high activity rate of three commits per day.
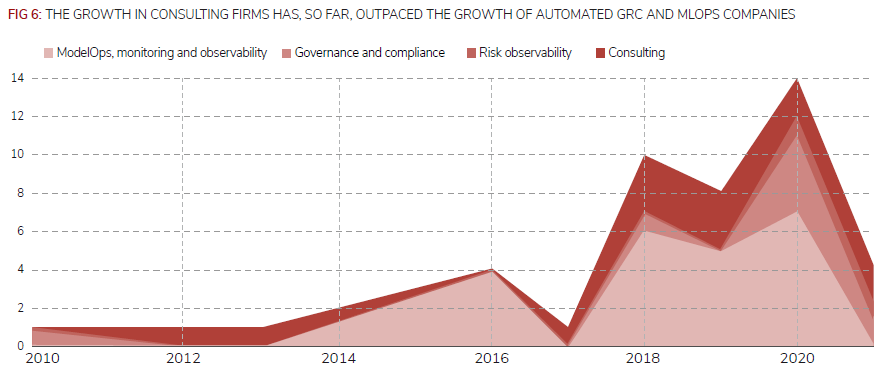